
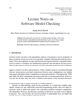
Continuous heart rate variation monitoring is an important measure to diagnose and prevent the fatal cardiovascular diseases. Due to the recent development of the wearable sensory devices, ballistocardiographic (BCG) records can be collected over a long period of time without the restrictions to the patient's normal activities. However, the physical activities of the patient may severely interfere with the BCG sampling and thus leading to the degrading of the signal quality. In this paper, we introduce a novel approach to recovering the beat-to-beat heart interval estimates by applying an unsupervised machine learning framework consisting of the bag of words model and the n-gram model. First, we adopt L1 and L2 norm minimization based linear filters to reveal the global signal patterns. Then we learn the cluster centers of the signal segments containing local peaks with a Gaussian mixture approach. N-gram model is applied to detect the repetitive pattern of the cluster centers within the signals, the learned heartbeat model acts as a further filter by the convolution with the time-varying signals. Finally, auto-correlation based interval prediction is used with the help of a Gaussian prior. The proposed approach is tested using the data collected from a number of subjects with different ages and genders. We compare the resulting estimates with the blood pressure oximeter references, and the experiments show that the proposed algorithm is able to provide reliable and accurate estimates with the standard error for the interval estimate of 12.2ms.