
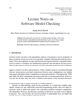
Information fusion from dissimilar sensors is best performed through extraction of attributes that can be measured by each of these sensors. In this way both imaging (Synthetic Aperture Radar (SAR), and Forward Looking Infra-Red (FLIR)) and non-imaging sensors (Identification Friend or Foe (IFF), Electronic Support Measures (ESM), radar) can be treated on an equal footing. In order to properly identify the target platform through repeated fusion of identity declarations, the attributes measured must be correlated with known platforms through a comprehensive a priori platform database. This comprehensive database is carefully analyzed for attributes that can be provided by sensors and additional knowledge that can be interpreted at all levels of fusion. The identity (ID) of the target platform can be provided in a hierarchical “tree” form where leaves are unique IDs but branch nodes correspond to a taxonomy obeying certain standards. In some cases, precise attribute measurement is either impractical or of moot value, so fuzzification is performed through appropriate membership functions. The actual identity of tracked ships is performed by an algorithm utilizing the Dempster-Shafer theory of evidence. The algorithm can mathematically handle conflict, which possibly appears as the result of countermeasures and/or poor associations, and ignorance, which may be present in the cases when sensors provide ambiguous or hard to interpret results. Since each imaging sensor has its own measurement potential, customized classifier solutions must be designed for optimal performance. A series of FLIR classifiers is presented and fused through a neural net fuser, while a hierarchical SAR classifier is shown to perform well for combatant ships, which are most likely to be imaged by the SAR. The complete fusion solution is demonstrated in a series of realistic scenarios involving both friendly and enemy ships.