
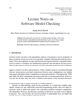
Recommendation models based on collaborative filtering have good prediction accuracy; however, they have very poor performance in cold-start scenarios, when no or few rating data are associated to certain items or users. An approach for dealing with cold-start problem is to build hybrid models that include content-based filtering. This paper focuses on knowledge-based hybrids that exploit semantic relations among item attributes to enhance traditional models. We investigate novel methods for exploiting this semantics during the recommendation process, in domains where explicit semantic relations among attributes in the form of ontologies are not available. We carry out an extensive performance comparison in which the proposed semantically-enhanced models are evaluated in normal and cold-start scenarios. The experimental results with a publicly available dataset demonstrate superior better performance of the proposed semantically-enhanced models, above all in cold-start scenarios, with respect to a state-of-the-art collaborative-filtering model based on matrix factorization. The proposed models, additionally, provide for interpretable recommendations, in contrast to black-box models.