
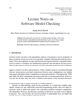
A significant risk following a kidney transplantation is graft loss. The Screen Reject Project has developed a Clinical Data Warehouse (CDWH) as a foundation for a clinical decision support system designed to improve the diagnosis of graft rejections. The CDWH integrates patient data and event records of n = 141 kidney transplant patients. These data are not directly comparable within the cohort as they consist of irregular time series, particularly of laboratory values. Therefore, a pre-processing routine was developed which divides a relative time window before the last biopsy (the relevant end event of the reference period for subsequent machine learning procedures) into equal time intervals for each patient. For each of these intervals a representative value is calculated from the contained laboratory values. These representative values are used to train models for predicting kidney rejection. The comparison with an existing study from the project, in which a classification model was developed without considering the temporal dependencies, shows an improved sensitivity and specificity in predicting kidney rejection for the harmonised data using the same random forest model.