
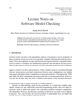
Digital pathology has made significant advances in tumor diagnosis and segmentation; however, image variability resulting from tissue preparation and digitization - referred to as domain shift - remains a significant challenge. Variations caused by heterogeneous scanners introduce color inconsistencies that negatively affect the performance of segmentation algorithms. To address this issue, we have developed a joint multitask U-net architecture trained for both segmentation and stain separation. This model isolates the stain matrix and stain density, allowing it to handle color variations and improve generalization across different scanners. On 180 stain images from three different scanners, our model achieved a Dice score of 0.898 and an Intersection Over Union (IoU) score of 0.816, outperforming conventional supervised learning methods by +1.5% and +2.5%, respectively. On external datasets containing images from six different scanners, our model averaged a Dice score and IoU of 0.792. By leveraging our novel approach to stain separation, we improved segmentation accuracy and generalization across diverse histopathological samples. These advances may pave the way for more reliable and consistent diagnostic tools for breast adenocarcinoma.