
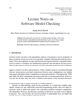
Multimorbidity is increasingly prevalent as the population ages and individuals with multiple long-term conditions (MLTCs) live longer. Often each condition is treated by a separate clinician, which can lead to harmful drug-drug and drug-disease interactions. Artificial Intelligence (AI) can help to identify those at risk of poor outcomes, which is particularly difficult when managing frail patients with MLTCs. We aim to analyze MLTC trajectories, but there is limited work on clustering using Electronic Health Records (EHR) and they largely ignore the time elapsed between clinical events. In this work we adapted three machine learning methods (Word2Vec, Autoencoder, TG-CNN) to allow us to cluster the entire trajectory. The proposed methods are tested on the ACT-MOOC dataset as a proof of concept, using timelines of interaction with an online course instead of an EHR. We find that clustering using the novel TG-CNN approach, which accounts for the time between events, shows a clear separation among different clusters and is better able to represent patient/user trajectories. In future work, we will apply this methodology within CPRD as part of the NIHR-funded DynAIRx project.