
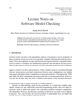
The goal of segmentation in abdominal imaging for emergency medicine is to accurately identify and delineate organs, as well as to detect and localize pathological areas. This precision is critical for rapid, informed decision-making in acute care scenarios. Vision foundation models, such as Segment Anything Model (SAM), have demonstrated remarkable results on many different segmentation tasks, but they perform poorly on medical images because of the scarcity of medical datasets. They lack robust generalizability across diverse medical imaging modalities, and they need to be fine-tuned specifically for medical images, as these images considerably differ from natural images. This study aims to investigate the application of a foundation segmentation model to ultrasound (US) images of the abdomen. We employed SAMed to segment and classify all organs and free fluid present in each US image. A dataset comprising 286 US images, corresponding segmentation masks, and organ-level labels was collected from the Bern University Hospital Inselspital. Due to the relatively small size of our dataset, we pre-trained SAMed on a larger public US dataset to fine-tune it for US imaging. We then applied this fine-tuned SAMed on the Inselspital dataset to generate multi-class masks and assessed its performance against ground truth annotations using standard evaluation metrics. The results demonstrated that the fine-tuned SAMed can identify and classify multiple organs, though challenging cases, such as free fluid segmentation, reveal opportunities for improvement. Furthermore, transfer learning proved to be a reliable solution for managing small datasets, a key obstacle in the medical imaging realm.