
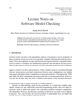
The stability of engineering structures will be greatly affected when they are subjected to stationary random loads. Such loads must be accurately identified to ensure the structural safety. Due to the complexity of stationary random loads and difficulty of solving inverse problems, traditional methods require improvement in identification accuracy. In this work, a stationary random load identification method based on ensemble empirical mode decomposition (EEMD) and deep recurrent neural network (RNN) is proposed. The method designs a deep RNN model consisting of one gated recurrent unit (GRU) layer, one bidirectional long short-term memory (BLSTM) layer, two long short-term memory (LSTM) layers, and two fully connected (FC) layers in sequence. The load and response signals are decomposed into a set of intrinsic mode functions (IMF) from high to low frequencies using EEMD, with the decomposed signals serving as the output and input of the network, respectively. The effectiveness of the proposed method is verified by the simulation data of a three-degree-of-freedom (3DOF) linear system, and the results show that the proposed method is with higher accuracy than using only deep RNN without signal decomposition. The method is verified by the experimental data of a clamped beam as well, with results showing a high accuracy of stationary random load identification.