
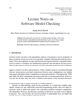
Atrial fibrillation (AF) is a prevalent cardiac arrhythmia associated with severe complications such as ischemic stroke and heart failure. Early detection is essential for timely intervention; however, traditional diagnostic methods often lack scalability and accessibility. This project explores the use of photoplethysmography (PPG) signals recorded via smartphone applications to develop a predictive neural network model for AF detection. Data collection involved student participants using a smartphone app, supplemented with curated open-source datasets to enhance generalizability. A multilayer perceptron (MLP) was designed and trained using TensorFlow, with model performance evaluated based on accuracy, sensitivity, specificity, and F1-score. The initial model achieved an accuracy of approximately 75%, indicating the potential of PPG-based AF detection. Optimization efforts continue, focusing on improving predictive accuracy and minimizing false positives. Future research will refine the model architecture, validate it on larger datasets, and integrate findings into scalable digital health initiatives, such as the Austrian Digital Heart Program, supported by the Austrian Institute of Technology (AIT).