
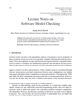
Introduction:
Predictive models hold significant potential in healthcare, but their adoption in clinical settings is hampered by limited trust due to their inability to recognize when presented with unfamiliar data. Estimating knowledge uncertainty (KU) can mitigate this issue. This study aims to assess the capabilities of two targeted approaches, specifically Ensemble Neural Networks (ENN) and Spectral Normalized Neural Gaussian Processes (SNGP), in quantifying KU and detecting out-of-distribution (OoD) data within the context of delirium risk prediction.
Methodology:
Using a cohort of hospitalized patients, the performance of ENN, SNGP, and a Random Forest (RF) baseline was evaluated in predicting delirium risk and identifying OoD data, which was generated through feature randomization and swapping techniques.
Results:
Both approaches achieved AUROC values (∼0.90) comparable to the RF baseline, with the added advantage of KU estimation. SNGP outperformed ENN, recognizing 82.4% and 92.2% of OoD samples compared to 68,8% and 86.0% in the feature-swapping and feature-randomization scenario.
Discussion:
While all models effectively predicted delirium risk, SNGP demonstrated superior performance in OoD detection, highlighting its potential to enhance reliability and trustworthiness of clinical decision support systems through robust KU estimation.