
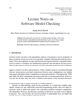
Background:
Managing geriatric risks is challenging, as early detection of conditions like delirium, frailty, dysphagia, falls, and malnutrition relies on time-intensive manual assessments requiring geriatric expertise. ML approaches can support healthcare professionals by enabling automated risk prediction using electronic health records (EHR) data, but existing models often lack geriatric-specific features.
Objectives:
This study examines whether incorporating geriatric-specific features improves EHR-based prediction models for key geriatric risks and whether a one-shot Federated Learning (FL) approach enhances performance.
Methods:
EHR data from three hospital providers were linked with acute geriatric data to develop and compare three model types: (1) a baseline model using only EHR data, (2) a model incorporating geriatric-specific features, and (3) a FL ensemble.
Results:
Inclusion of geriatric-specific features significantly improved model performance, while a one-shot FL approach provided only marginal, non-significant additional benefit.
Conclusion:
Geriatric-specific features are essential for capturing the complexity of the acute geriatric population.