
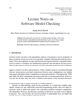
Background:
Type 2 diabetes (T2D) continues to present a global public health challenge due to its increasing prevalence. Early diagnosis is critical for preventing complications, but current screening methods often fail to detect early diabetic conditions.
Objectives:
This study aimed to classify T2D patients from healthy individuals using high-resolution N-glycan profiling.
Methods:
Glycan profiling was performed on serum samples from 161 individuals using capillary electrophoresis with laser-induced fluorescence detection. Different classification methods were fine-tuned using hyperparameter optimization and feature selection techniques, and their performance was comprehensively evaluated based on quality metrics.
Results:
The Extra Trees Classifier outperformed the other models with the highest median AUC, demonstrating robust accuracy (0.8982), sensitivity (0.8966), and specificity (0.9000).
Conclusion:
N-glycan profiling combined with machine learning provides a promising approach for early T2D detection. The Extra Trees Classifier showed exceptional predictive performance, warranting further investigation with larger datasets to validate its clinical applicability.