
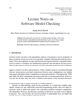
Background:
The accumulation of Real-World Data (RWD) from Electronic Health Records (EHRs) and registries offers substantial potential for generating Real-World Evidence (RWE). However, the ability to generate robust evidence from real-world data hinges on its quality. This is especially critical when heterogeneous data is first transformed into standardized, research-ready data models.
Objective:
This study presents an approach for assessing data completeness through a pipeline for extracting and transforming oncological RWD.
Methods:
We introduce a technical solution that enables the assessment of data completeness across three data transformation stages, beginning with the initial data source and extending through Health Level 7 (HL7) Fast Healthcare Interoperability Resources (FHIR) to CSV.
Results:
Using Trino, a distributed SQL engine, we evaluate data completeness at the three transformation stages by comparing cancer diagnosis counts. The modular pipeline design, compatible with various data sources, allows for error detection in ETL processes.
Conclusion:
Future work will expand the system to address additional data quality dimensions, such as correctness and plausibility, improving the overall robustness of data analytics in federated environments.