
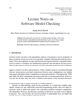
Evaluating the blood smear test images remains the main route of detecting the type of leukaemia, accurate diagnosis is fundamental in providing effective treatment. The changes in the structure of the white blood cells present different morphological characteristics translated into extractable features. This paper explores techniques for manipulating a reduced dataset to increase the classification with CNN (Convolutional neural Network) and feature extraction. Extracting ROI (Regions of Interest) divides the leukaemia images into points of interest respective white blood cells, expanding the dataset an important factor for CNN’s performance. Segmenting the initial dataset into ROI through computation after applying Otsu thresholding results in a new dataset of images. The two datasets are analysed, feature extraction performs better on the initial dataset while CNN’s accuracy is higher for ROI images. Further steps will divide the images into filtered regions of interest where more specific characteristics are extracted to increase the accuracy.