
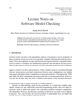
This paper introduces a novel approach for predicting symptom escalation in chemotherapy patients by leveraging Convolutional Neural Networks (CNNs). Accurate forecasting of symptom escalation is crucial in cancer care, as it enables timely interventions and enhances symptom management, ultimately improving patients’ quality of life during treatment. The analytical dataset consists of daily self-reported symptom logs from chemotherapy patients, capturing a variety of symptoms such as nausea, fatigue, and pain. However, the data was significantly imbalanced, with approximately 84% of entries showing no symptom escalation. To address this issue and enhance the model’s ability to identify symptom escalation, the data was resampled into varying interval lengths, ranging from 3 to 7 days. This resampling allows the model to detect notable changes in symptom severity over different time frames. The study’s results show that shorter intervals (3 days) delivered the best performance, achieving an accuracy of 79%, a precision of 85%, a recall of 79%, and an F1 score of 82%. As the interval length increased, both accuracy and recall declined, though precision remained relatively consistent. These findings illustrate the capability of CNN-based models to capture temporal patterns in symptom progression effectively. Incorporating such predictive models into digital health platforms could empower healthcare providers to offer more personalized, proactive care, allowing for earlier interventions that may reduce symptom severity and improve adherence to treatment