
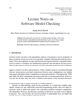
In recent years, Knowledge Graphs (KGs) have sprung up in various fields to support a variety of applications, like question answering, recommendations, etc. When integrating knowledge from different KGs, a common task is to identify some equivalent entities from multiple KGs describing real-world objects, which is called the entity Alignment (EA) task. In recent years, unsupervised EA methods have attracted the interest of many researchers and achieved many research results. However, there are few literatures that comprehensively compare and analyze the modeling frameworks, research ideas and techniques of these methods. This chapter focuses on the research ideas and status of this field in order to provide practical insights. We summarize and analyze 22 unsupervised EA methods that have emerged in recent years, and propose a general framework for unsupervised EA methods. Then the related methods are divided into two categories: EA methods with training and EA methods without training, and the characteristics and implementation techniques of the related methods are analyzed. In addition, the current research challenges in this field are summarized and future research directions are prospected.