
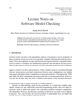
Transformer-based language models are known to encode factual information in their hidden representations, however, an open question is how to explicate this implicitly encoded knowledge. Approaches to unveil encoded factual associations relate to prompt-based methods, techniques to localize or edit specific model weights, and to trace the information flow during inference. Inspired by evidence of entire dependency graphs in embedding (sub-)spaces of these models, this chapter presents an approach to utilize linear transformation of a model’s vector representation space to uncover the properties of knowledge graphs. Subjects and objects of factual knowledge graph triples, i.e., from Wikidata, are expected to be near neighbors, a proximity of knowledge entity embeddings that can be measured with simple cosine similarity and approximated by training a transformation matrix in a supervised manner on minimizing the distance between the actual and predicted similarity scores. The obtained results are promising in terms of Pearson and Spearman correlations as well as mean squared error rates, indicating that there is a specific vector sub-space that encodes factual associations in the last layer of neural language models.