
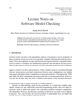
Graph representation learning has garnered significant attention due to its outstanding performance across numerous real-world applications, such as social network analysis, bioinformatics, and recommendation systems. However, supervised graph representation learning models often struggle with label sparsity, as data labeling is time-consuming and resource-intensive. To address this, few-shot learning on graphs (FSLG) has been proposed, combining the strengths of graph representation learning and few-shot learning to mitigate performance issues caused by limited annotated data. This chapter comprehensively presents the body of work in FSLG. It begins by introducing the challenges and foundational concepts of FSLG. The chapter categorizes and summarizes existing FSLG research into three major graph mining tasks at different granularity levels: node, edge, and graph. Node-level tasks involve predicting labels for individual nodes, edge-level tasks focus on predicting relationships between nodes, and graph-level tasks involve predicting properties of entire graphs. This organization provides a clear overview of various methodologies and applications in FSLG. Finally, the chapter discusses potential future research directions in FSLG, which aims to inspire further investigation and innovation in FSLG, advancing its development and application to create more effective AI solutions.