
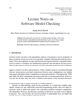
In the modern era of image-based applications, efficient and accurate image search algorithms have become essential. This study presents an innovative method to improve scene picture search by applying metric learning neural networks on a Neural Network Selection (NNS) architecture. The fundamental goal is to acquire relevant images that capture semantic similarities between scenes, resulting in improved retrieval performance with adequate implementation with compact PCs such as a Raspberry Pi. Metric learning is applied to determine the metrics of image similarity based on the feature extracted by a neural network. Based mainly on the quality of feature extracted, the proposed NNS architecture will select the best neural model using an evaluation criteria, to calculate the selection score. This formula considers accuracy, precision, recall, device, power consumption, and response time, evaluating the model’s computation efficiency. In the experiment, an autoencoder on the neural network selection architecture is tested using an ECG dataset with 5,000 data points. The Mean Squared Error (MSE) is used to quantify the autoencoder’s performance. A suitable MSE threshold is established, and data points with errors over the threshold are considered anomalies, while those below the threshold are considered normal. Performance on this anomaly detection results in accuracy worth 0.942, precision worth 0.994, and recall worth 0.901. Neural network selection can also apply these values in addition to MSE to calculate the selection score for selecting the best neural model.