
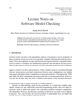
In this paper, a dynamic curation method that uses pretrained models of art interpretation is proposed. The proposed method leverages exhibition catalogs (primary classifications and images) as a source of curatorial knowledge to construct a machine learning model, thereby using this model to evaluate, position, and visualize other artwork. This method enables the dynamic curation of artwork in a vast integrated art collection archive. A prototype system for the proposed method was implemented and applied to two exhibition catalogs at the Artizon Museum. In the experiment, artworks matching the purpose and art style of the modeled exhibition from the archives of the Metropolitan Museum of Art in New York City and Paris Musées were evaluated using the constructed model. The experiment demonstrated that the proposed method successfully classifies and enables the visualization of artwork and images available in the open data from the MET and Paris Musées in alignment with the intended theme of the exhibition for which the model was constructed. Feedback from curators and art professionals indicates that the proposed method can be used to compare museums with art collections of the same genre and to organize new exhibitions based on the curatorial model.