
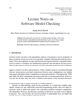
This paper proposes an innovative method for knowledge construction and application based on the concept of space mapping. Space mapping is a mathematical operation that maps a vector to a space, expressing the relationship between input and output information. By representing data as vectors and matrices, the method establishes a mathematical relationship between input and output using a “knowledge vector”. The “dark-matter matrix” transforms the input vector into the knowledge vector, mapping the input data onto the output space. The approach is extended to “parallel spaces”, allowing for independent knowledge vectors in each space.
The paper defines concepts related to space mapping, such as space-time mapping, time-space mapping, chain mapping, and parallel spaces. It presents schemes and methods for knowledge construction and application based on space mapping processing, including vector construction techniques and knowledge modeling methods like chain mapping and parallel spaces. These techniques enable handling complex data and implementing efficient, scalable knowledge construction and application schemes.
The method is illustrated with two application examples: robot navigation in a maze and image recognition using chain mapping. The results demonstrate the method’s ability to handle temporal, high-dimensional, and heterogeneous data aspects, create non-redundant knowledge vectors, and generate different output information based on application requirements.