
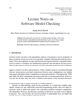
In the contemporary era, with its incidence rising at an alarming rate, diabetes has become a major global health concern. This work presents a data mining approach that compares the K-Means and Fuzzy C-Means (FCM) clustering algorithms to answer the increasing demand for accurate diabetes management and prediction in a wide range of datasets. As data mining is essential for drawing insightful conclusions from large, complicated datasets, the study concentrates on using these methods to improve the precision and effectiveness of diabetes prediction models. The diabetes dataset is divided into separate clusters using FCM and K-Means after preprocessing steps to manage missing values and outliers. Algorithms often define the clustering process’s conclusion and the effectiveness of its domain application. Two significant clustering algorithms centroid-based K-Means and representative object-based FCM (Fuzzy C-Means) clustering algorithms are compared in this research work. These techniques are used, and the effectiveness of the output clustering is to assess performance. The evaluation metrics accuracy, sensitivity, specificity, and AUC-ROC highlight the model’s superior performance over individual clustering techniques. This work addresses the urgent problem of diabetes currently, which advances the field of diabetes prediction and highlights the crucial role of data mining, which plays in healthcare analytics over early detection and intervention.