
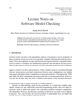
Globally, skin cancer—especially melanoma—is a major health concern, and better patient outcomes depend on early and precise diagnosis. Manual examination is a common component of traditional diagnostic techniques, but it is labor-intensive and subject to error. Promising approaches to automate skin lesion analysis have been made possible by recent developments in deep learning. In order to overcome the shortcomings of current approaches, this work investigates the creation of a hybrid deep learning model that blends VGG16 and ResNet50 architectures. The suggested approach incorporates sophisticated preprocessing methods, such as picture normalization and data augmentation, to improve feature extraction and classification accuracy using datasets like ISIC 2018 and HAM10000.Important results show that the hybrid model outperforms state-of-the-art benchmarks in performance metrics, achieving 98.75% training accuracy and 97.50% validation accuracy. The model’s enhanced precision (97.60%), recall (97.55%), and F1 score (97.58%) highlight how reliable it is at differentiating between benign and malignant tumors. The study also emphasizes how crucial it is to strike a balance between model complexity and computing performance in order to support practical clinical deployment. This study advances computer-aided diagnostic (CAD) systems for the identification of skin cancer by tackling issues including class imbalance and dataset unpredictability. The suggested method has a great deal of promise to improve early diagnosis, lessen the need for invasive treatments, and assist dermatologists in making clinical decisions. This paper thoroughly reviews current methodologies used in skin cancer detection, offering a timely resource for researchers developing automated and precise melanoma detection models.