
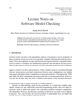
With the rapid expansion of industrial IoT (IIoT), maintaining robust cybersecurity is essential for the smooth operation of industrial processes. Industrial environments require adaptive solutions to effectively mitigate evolving cyber threats and protect sensitive operations. This research aims to improve the cybersecurity of industrial IoT environments. The research intends to design and implement an adaptive and real-time intrusion detection system with edge computing integration that improves the reliability of the operations in industrial IoT. We incorporated machine learning approaches to classify cyber threats using XGBoost and Deep Neural Networks (DNN). A comparative analysis of results obtained from two datasets shows that the XGBoost model was slightly more accurate than the DNN model, with an accuracy of 79% for dataset D1 and approximately 99.42% for data set D2. This analysis also clearly demonstrates the usefulness of these machine learning approaches and the need to select a model depending on the requirements for detecting particular attacks. Confusion matrix analysis shows that both models have several advantages in terms of recognizing different types of cyber threats.