
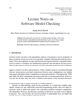
The usage of Machine Learning in the clinical domain enables better decision-making skills and improves the quality of health care services rendered to the patients. This paper described a new MLOps framework which addresses the challenges faced when applying AI in the clinical domain. These include the handling of heterogenous, incomplete and evolving medical data sets, ensuring privacy of patients, controlling the versions of dataset and models adopted. The proposed framework supports a repository of evolving anonymized patient medical data, tracking of the feature selection and models adopted using versioning control system and records the accuracy of their outcomes. It also enables audit tracking and ensures transparency and accountability, thus helping to increase medical workers’ confidence in Machine Learning and encourages the use of ML in the clinical work. For evaluation, the proposed framework is set up in the clinical environment using existing patient’s records. Evidences, such as records of model training using specific datasets traceable to the actual patients’ records, are collected. These evidences fulfill the audit requirements and form the basis to justify research funding in future AI-related medical work. Finally, the study also proposes future work and directions to extend the framework into other domains.