
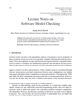
This research aims to develop an advanced material detection system for conveyor belts, utilizing state-of-the-art image processing and machine learning techniques to automate the identification of various materials, thereby enhancing operational efficiency and accuracy in industrial settings. Current methods in material detection, such as traditional manual sorting and basic automated systems, often need more precision and adaptability in dynamic industrial environments. This paper identifies a gap in the practical and reliable detection of diverse materials under varying environmental conditions. The primary research questions addressed include: How can modern image processing and machine learning techniques improve material detection accuracy? What optimizations can be applied to ensure real-time processing efficiency? The proposed solution integrates a strategic camera setup with controlled lighting, robust image preprocessing algorithms (noise reduction, normalization, and resizing), and custom-designed detection algorithms using machine learning models. This system outperforms existing solutions by offering higher detection accuracy and adaptability to diverse industrial conditions. An interface is developed to display detection results and provide intuitive controls for system adjustments, ensuring practical use by industrial professionals. Rigorous testing and validation processes are implemented to enhance detection accuracy and processing speeds, with specific performance metrics established to measure efficacy. This paper provides a transformative impact on the manufacturing and processing industries by addressing environmental variability and material diversity.