
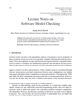
This research delves into the comparative assessment of diverse deep learning architectures for the automated identification of pulmonary diseases in chest X-ray images, aligning with the diagnostic framework of the International Classification of Deseases, 10th Revision (ICD-10). The evaluated architectures encompass Convolutional Neural Network (CNN), MobileNetV2, DenseNet, VGG16, and InceptionV3. Through an extensive investigation conducted on a comprehensive dataset comprising 108,948 chest X-ray images, we scrutinize the performance metrics and diagnostic accuracy of each architecture. Notably, our findings reveal MobileNetV2 as the frontrunner, exhibiting an outstanding accuracy rate of 97% for Emphysema disease. This remarkable accuracy underscores the robustness and efficiency of MobileNetV2, even amidst large- scale diasets, positioning it as a promising solution for the automated detection of pulmonary ailments from medical imaging. Additionally, we analyze the computational efficiency and resource requirements of each architecture, providing insights into their practical applicability in real-world clinical settings. The implications of our study extend beyond technical benchmarks, offering valuable insights for healthcare practitioners, researchers, and developers working towards enhancing diagnostic processes in pulmonary healthcare. By elucidating the comparative strengths and limitations of various deep learning architectures, this research contributes to the advancement of computer-aided diagnosis systems, paving the way for more accurate and efficient detection of lung diseases from chest X-ray imaging data.