
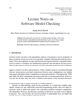
This paper considers symbol grounding in its practical and theoretical aspects. Taking up the theoretical perspective, we begin by considering the relative inefficiency of large language models in acquiring language. A framework is introduced based on the concept of morphological computation and formalised with reference to conditional Kolmogorov complexity: that the form of embodied experience scaffolds human language acquisition. This argument is extended to consider the symbol grounding problem, with particular reference to the origin of language in both the individual and historical sense. It is argued that, while humans also make use of statistical learning, the process of symbol grounding via morphological computation is essential at the origins of language and during early development. It provides a minimal ontology in terms of objects, containers, processes, etc.—basic features which language models must instead brute force by statistical means. The paper closes by reconsidering the symbol grounding problem in light of recent advances, particularly the promise of multi-modal models and robotics, and ultimately concludes that the status of the symbol grounding problem depends upon our aims in the pursuit of artificial intelligence.