
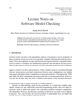
Defect detection of substation equipment components is an indispensable part of grid security situational awareness, and the regular inspection of equipment is related to the secure operation of the power system. For the current problem of low recognition accuracy of the defect classification model of substation equipment components, this paper proposes a defect classification method based on the improved Dilated Convolution Swin Transformer (DC-Swin). First, an improved Dilated Convolution Self-Attention Module is constructed for extracting the regions of equipment components that contain rich defect-specific information; Secondly, this paper constructs an image dataset using infrared imaging of defects of equipment components to pre-train the module, which enables the Self-Attention Module to learn the important regions in the image and reduces other ineffective information interfering with the model; Finally, this paper incorporates pre-trained modules, which have undergone preliminary training on flawed infrared imaging datasets, with the Swin Transformer, specifically in the channel dimension. By seamlessly integrating crucial feature regions into the original image, the network model is empowered to delve deeper into the intricate dependencies among various features, resulting in an enhanced and more discriminative feature representation capability. The proposed method improves the accuracy by 6.17% compared to the original Swin Transformer model. The results show that the method achieves the optimal utilization of the defect classification model on the acquired dataset and provides a solid foundation for substation safety situational awareness.