
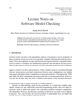
To improve the accuracy of power load forecasting, a short-term load forecasting method based on Principal Component Analysis (PCA), XGBoost (eXtreme boosting system), and Long Short Term Memory (LSTM) using neural networks is proposed. By studying the variation patterns of residential electricity load, a feature set is constructed using date factors, climate factors, and daily load factors as inputs. Firstly, the sample dataset is divided into a load dataset and an influencing factor dataset composed of date and climate factors for data preprocessing; Then, the PCA principal component analysis method is used to extract features with significant impact, and a strong correlation feature vector is formed with the load data. The results are input into XGBoost and LSTM networks for mathematical fusion; Finally, using the electricity load data of residents in a certain area of Nanjing, the parameters of each group of network models were trained and the load prediction values were output. The results were compared and verified with the Convolutional Neural Networks (CNN) model, LSTM network model, the Gated Recurrent Neural Network (GRU) model and XGBoost LSTM network model, respectively. The results showed that the method can effectively reduce the error between the predicted values and the true values.