
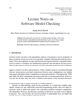
Machine Learning (ML) models often operate as black-boxes, lacking transparency in their decision-making processes. Explainable Artificial Intelligence (XAI) aims to address the rationale behind these decisions, thereby enhancing the trustworthiness of ML models. In this paper, we propose an extension of the Explainable ML Workflows ontology, which was designed as a reference ontology with OntoUML, and implemented as an operational ontology with OWL. The Explainable ML workflows ontology reuses ML-Schema, which is a core ontology for representing ML algorithms. We have identified four main issues in the conceptualization of this ontology, namely the lack of feature categorization, the lack of data pre-processing methods, the shallow description of metadata related to training and testing, and the lack of detailed representation of XAI approaches and metrics. We addressed these four issues in the so-called Explainable ML Pipeline Ontology (XMLPO), which aims to provide a comprehensive description of the ML pipeline for XAI. XMLPO offers a deeper understanding of the entire ML pipeline, encompassing data input, pre-processing, model training and testing, and explanation processes. XMLPO was validated through a case study on the prediction of specific performance indicators in a manufacturing company, and the results of this validation showed that the ontology helps data scientists to better comprehend a ML pipeline and the features that influence the ML prediction model the most.