
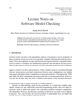
Situationally-aware artificial agents operating with competence in natural environments face several challenges: spatial awareness, object affordance detection, dynamic changes and unpredictability. A critical challenge is the agent’s ability to identify and monitor environmental elements pertinent to its objectives. Our research introduces a neurosymbolic modular architecture for reactive robotics. Our system combines a neural component performing object recognition over the environment and image processing techniques such as optical flow, with symbolic representation and reasoning. The reasoning system is grounded in the embodied cognition paradigm, via integrating image schematic knowledge in an ontological structure. The ontology is operatively used to create queries for the perception system, decide on actions, and infer entities’ capabilities derived from perceptual data. The combination of reasoning and image processing allows the agent to focus its perception for normal operation as well as discover new concepts for parts of objects involved in particular interactions. The discovered concepts allow the robot to autonomously acquire training data and adjust its subsymbolic perception to recognize the parts, as well as making planning for more complex tasks feasible by focusing search on those relevant object parts. We demonstrate our approach in a simulated world, in which an agent learns to recognize parts of objects involved in support relations. While the agent has no concept of handle initially, by observing examples of supported objects hanging from a hook it learns to recognize the parts involved in establishing support and becomes able to plan the establishment/destruction of the support relation. This underscores the agent’s capability to expand its knowledge through observation in a systematic way, and illustrates the potential of combining deep reasoning with reactive robotics in dynamic settings.