
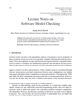
As Unmanned Aerial Vehicles (UAVs) become integral to urban infrastructure, their ability to communicate effectively with human operators and adapt to dynamic environments is crucial. This paper presents an innovative approach to enhancing UAV performance in transportation and traffic monitoring by integrating emotional intelligence through the PAD (Pleasure, Arousal, Dominance) model. The proposed system architecture includes a comprehensive data collection layer that gathers diverse inputs from sensors and contextual information, a perception analysis layer that processes these inputs to generate emotional states using the PAD model, and a response layer that translates these emotional states into specific behaviors through behavioral mapping and adaptation modules. Detailed methodologies, including pseudocode and flowcharts for key modules such as data normalization, PAD calculation, mood updating, and mood octant determination, are provided for clarity and reusability. The system’s effectiveness is validated through practical scenarios such as routine surveillance, heavy traffic monitoring, and incident detection, demonstrating significant improvements in UAV adaptability and interaction. Key contributions include the development of a multi-dimensional emotional model for UAVs, a dynamic mood updater module, and the successful application of the PAD model in complex traffic monitoring scenarios. This approach significantly enhances UAV performance, ensuring more natural interactions with human operators and better adaptability to real-time traffic conditions. It paves the way for future exploration into emotionally intelligent autonomous UAV systems.