
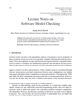
The process of urbanization on a global scale has generated a significant increase in metropolitan populations, which in turn brings with it a series of challenges for the management of transport infrastructure. In this context, the importance of accurate traffic flow prediction in urban traffic management systems stands out, with the city of Catania being used as the focus of this study. However, predicting traffic flow faces challenges, such as the high costs associated with acquiring extensive real traffic data, particularly when installing sensors on every road is not feasible. To address the issue of missing traffic flow data on roads without sensors, this study proposes an innovative approach based on machine learning, using the Long Short-Term Memory architecture and incorporating both sensor and floating car data. The results obtained are thoroughly analyzed and discussed, highlighting the ability of the LSTM model to provide accurate predictions of urban traffic, even with a limited amount of data available. These findings highlight the relevance of employing advanced machine learning techniques in the efficient management of urban mobility, aiming to improve the quality of life in cities and deal more effectively with the challenges of contemporary urban traffic.