
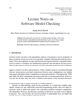
Traffic congestion has been a major concern in urban areas due to its strong impact on various social, economic, and human safety sectors. Understanding the relationship and analyzing the trends and patterns between congestion and accidents can strengthen the strategy for reducing traffic congestion. Research on causes of accidents and their impact on congestion has recently been explored on a greater scale, but there is still a lot of scope for vast areas of improvement. To tackle this issue, we built a Bayesian Network (BN) model for analyzing and predicting congestion probability that can occur due to accidents. In this work, the complexity of handling real data obtained from Darmstadt city is described in detail. The accidents and congestion are correlated by introducing a novel threshold-based approach, which identifies congestion based on the change in vehicle density immediately following an accident. Different thresholds are explored to determine the most reliable measure of congestion, with the T4 threshold emerging as the optimal choice. Moreover, the proposed BN model is evaluated against several machine learning models, demonstrating competitive performance and its ability to understand the root cause of traffic congestion.