
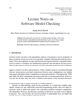
Roads are considered the artery of countries that feed cities and regions and have great importance in achieving high levels of services for its users in facilitating their transportation and their goods from one place to another. Governments attach increasing importance to road conditions to achieve sustainability in the services provided to roadway users and thus increase users’ satisfaction with government services. Perhaps one of the things that governments aim to monitor the road surface conditions. Where, Keeping the pavement in high-quality conditions can achieve the highest levels of comfort while driving, reducing vehicle maintenance costs, reducing traffic accidents, and thus increasing traffic safety. Monitoring the pavement condition is considered one of the significant applications to evaluate the pavement condition by determining the type and severity of defects on the pavement surfaces. In this study, a vibration-based method is used to measure the level of comfort riding a passenger car at a speed of 40 km/hr on local roads in Melbourne. The vibration signals are measured using an accelerometer sensor fixed on the front dashboard of a sedan car. The vibration signals then are smoothed and filtered to be used for the detection of the pavement defect locations according to the fluctuations in signals. Besides, the filtered vibration data was then divided into training and testing databases to develop an artificial neural network model named Long-Short-term memory LSTM. The LSTM is used to automatically detect and classify the quantity and quality of pavement defects according to the conducted vibration data.