
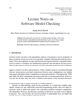
The development of autonomous vehicles (AVs) requires a comprehensive understanding of both explicit and implicit traffic rules to ensure legal compliance and safety. While explicit traffic laws are well-defined in statutes and regulations, implicit rules derived from judicial interpretations and case law are more nuanced and challenging to extract. This research investigates the potential of Large Language Models (LLMs), particularly GPT-4o, in automating the extraction of implicit traffic rules from judicial decisions. By utilizing various prompt engineering techniques, including Standard Prompts, Chain-of-Thought (CoT), Chain-of-Instructions (CoI), and Layer-of-Thought (LoT) prompts, this study aims to assess the effectiveness of GPT-4o in identifying normative content relevant to specific traffic laws. The contributions of this paper include an assessment of LLMs for legal text processing, the automation of implicit rule extraction, and the development of a scalable framework that can continuously update as new legal precedents emerge. The results indicate promising avenues for integrating automated normative extraction in AV systems, improving both the safety and legal compliance of autonomous driving technologies.