
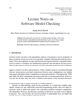
This study explores sentiment analysis research framework based on the Mandarin social media dataset, focusing on the Transformer model. The paper first reviews the background of sentiment analysis, emphasizing the importance of this task in text classification and the relative lack of sentiment analysis in Mandarin Chinese. The study used three public datasets from the CSDN platform, including positive and negative reviews from different social media platform, explores how word order impacts sentiment classification in Mandarin. The study completed the experiment through four stages: preprocessing, text embedding, feature extraction, and sentiment classification, and used a pre-trained Transformer model for analysis. The results demonstrate the effectiveness of Transformer models for sentiment analysis with high accuracy on certain datasets, although challenges persist with specific data due to complexity. Future work aims to refine the model’s performance on diverse datasets and address limitations in sentiment feature extraction for Mandarin texts. The results confirm that there is still much room for improvement in Transformer models in improving sentiment classification in non-English languages such as Mandarin.