
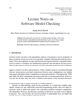
Hull resistance is a critical factor in determining a vessel’s performance, fuel consumption, and overall efficiency. Traditional methods for evaluating hull resistance involve time-consuming and expensive experiments or computational fluid dynamics (CFD) simulations.
Machine learning (ML) offers a promising alternative for predicting ship resistance, leveraging data-driven models to establish relationships between hull characteristics and resistance values. This study investigates the application of machine learning to evaluate a sailing yacht bare hull resistance using a machine learning Gaussian Process Regression model trained with the Delft systematic hull series. A dataset comprising 50 sailing yacht hull forms and corresponding resistance values evaluated for Froude numbers between 0.1 and 0.6 is used to train and validate various ML models. The models are evaluated based on RMSE, R-Squared and MSE values. The results demonstrate the effectiveness of ML Gaussian Process Regression models in predicting ship resistance and the can be integrated into the early stages of ship design, facilitating the optimization of hull forms for improved hydrodynamic performance.
In conclusion, this research establishes machine learning as a powerful tool for evaluating sailing yacht resistance, offering generalizable predictions that significantly contribute to more efficient, cost-effective yacht and ship design optimization.