
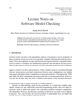
Multiple sclerosis (MS) is a complex neurodegenerative disease with a variable prognosis that complicates effective management and treatment. This study leverages machine learning (ML) to enhance the understanding of disease progression and uncover gender-based differences in MS by analyzing clinical data integrated with patient-reported outcomes (PROMs). We conducted a prospective cohort study involving 250 MS patients at a secondary care hospital in Spain over an 18-month period. Using REDCap for data management, we collected comprehensive demographic, clinical, and PROMs data. Our analysis utilized Decision Trees, Random Forest, and Support Vector Machine algorithms to classify patients based on disease evolution and infer Expanded Disability Status Scale (EDSS) levels. Additionally, we employed propensity score matching to analyze gender differences, focusing on clinical outcomes and quality of life measures. The results could indicate that integrating diverse data sets through ML would significantly improve the diagnostic accuracy and serve as a support for clinician’s decision making. Our models achieved high accuracy in classifying MS types and predicting disability levels, demonstrating the potential of ML in personalized treatment planning. Furthermore, our findings suggest notable gender differences in disease progression and response to treatment. These insights advocate for a gender-specific approach in MS management and highlight the importance of personalized medicine. This study underscores the transformative potential of ML in enhancing the understanding and management of MS through integrated data analysis.