
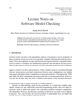
In order to realize the desire of all parties involved in instant retail, it can better understand the behavior of users, discover the hidden interests of users and the behavior rules of group users, and an intelligent recommendation system for instant retail services based on machine learning is proposed. Analyze and process the relevant data of e-commerce websites, use the improved rough set attribute reduction algorithm and Apriori improved algorithm for data mining, use the two improved algorithms together as a scheme to apply to the recommendation system, and then compare the recommendation efficiency of the system through empirical analysis of relevant data. The experimental results show that the time to generate frequent item sets based on the improved Apriori algorithm is significantly less than that based on the Apriori algorithm when the minimum support is fixed. In terms of the efficiency of generating frequent item sets, the Apriori improved algorithm is more efficient. With the minimum support getting smaller and smaller, the comparison becomes more obvious. When the number of transactions is fixed, the time used by the recommendation system to apply Scheme II is obviously less than that used by Scheme I. Moreover, with the continuous increase of the number of transactions in the transaction database, the difference in the time used to apply the two schemes increases significantly. In terms of recommendation efficiency of instant retail intelligent recommendation system, Scheme II is better than Scheme I.
Conclusion:
The system effectively improves the recommendation efficiency of the recommendation system.