
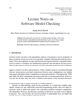
In order to solve the constraints on flexibility and scalability of traditional rule-based assessment methods in the comprehensive processing of massive, multi-dimensional and heterogeneous tax-related data for tax risk analysis of large enterprises, an intelligent prediction model for enterprise tax risk is proposed. Aiming at the typical scenarios of tax risk of related transactions of large enterprises, the characterization extraction method of tax data features is defined, and the tax risk analysis and prediction model based on artificial neural network multilayer perceptron is constructed. In order to evaluate the performance of the proposed model, a test dataset is constructed using real tax data and expert labeled data, and experiments are conducted under different positive and negative sample ratios and sample capacity sizes, and compared with several widely used machine learning models. The experimental results show that the model in this paper achieves better performance than the comparative models in both positive and negative sample balanced and unbalanced cases. Among them, when the ratio of positive and negative samples is 5:5, all the models achieve the optimal results relative to the data imbalance, while the model in this paper outperforms all the comparative models, and achieves the best precision rate, recall rate, F1 value and AUC value.
Conclusion:
The proposed method has good performance in terms of accuracy and effectiveness. With the continuous improvement of data labeling in tax authorities, the method of artificial neural network for tax risk assessment has a broad prospect in business use.