
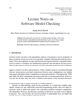
In order to solve the problem that text feature mining is insufficient due to the single feature word vector input form at the coding end, which further affects the extraction of text semantic information by the neural network model, the analysis of long text summary generation technology based on deep learning is proposed. This paper introduces the word frequency feature and semantic feature of words, and integrates the TF-IDF value of feature words and the semantic value obtained by LSA through naive Bayesian formula to form a new word vector. It effectively integrates the multi-dimensional features of the text, improves the ability to understand the meaning of words, and helps the encoder to learn the text information. In order to solve the problem that the traditional recurrent neural network has insufficient ability to encode long text and inaccurate acquisition of intermediate semantics, a deep proxy communication mechanism is introduced. The encoder with this structure can obtain global information more accurately and improve the ability to understand text. The experimental results show that each evaluation index has achieved good results. Compared with the benchmark generative summary method point generator+coverage, the automatic summary generation model LTSGDL in this paper has improved about 2.2% on the three summary evaluation indexes.
Conclusion:
Compared with the comparison method, the summary generated by this model has higher accuracy.