
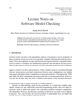
The ability to fulfil one’s responsibilities both at the workplace and at home while still finding time for interest and hobbies on a personal level is known as work-life balance. There is a need to implement intelligent technologies for shaping work-life balance, as physical, mental, and emotional well-being can all be enhanced by striking a better work-life balance. The need for more transparent and understandable models is growing along with our reliance on intelligent technologies. Furthermore, the current gold standard for establishing credibility and using AI in vital fields is the capacity to explain the model in general. This research seeks to employ XAI to comprehend, illustrate, and elucidate the findings derived from utilizing several machine learning models to determine the primary factors impacting the work-life balance of individuals in the private sector. The support vector machines (SVMs), random forests, and tree-based classifiers from XGBoost were trained and tested on a work-life balance dataset collected from private sector employees. Among all the models, the SVM provides the highest accuracy values for all the features of the feature set compared to the other two algorithms. The attribute importance for the best feature (working style) of the SVM model is explained using the feature importance plot, summary plot, and heatmap from the explainable artificial intelligence models SHAP and LIME. This comprehension makes it easier for decision makers and human resources (HR) practitioners to comprehend the model’s predictions and to implement initiatives that support each employee’s demand for a healthy balance between work and personal life.