
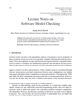
Indirect reciprocity (IR) is a key mechanism to explain cooperation in human populations. With IR, individuals acquire reputations which can be used by others when deciding to cooperate or defect: the costs of cooperation can therefore be outweighed by the long-term benefits of keeping a good reputation. Although IR has been studied assuming populations fully composed of humans, social interactions nowadays involve the ever-increasing presence of artificial agents (AAs) such as social bots, conversational agents or even collaborative robots. It remains unclear how IR dynamics will be affected once artificial agents co-exist with humans. Here we develop a theoretical model to investigate the potential effect of AAs, deployed with a fixed strategy, in the evolving cooperation levels observed in a population. We study settings where AAs are subject to the same reputation update rules as the remaining adaptive agents and settings where AAs have a fixed reputation. We show that introducing a small fraction of AAs with a discriminating strategy (i.e., cooperate only with good agents) increases the cooperation rate in the whole population. Moreover, the positive effect of AAs is exacerbated when these are unconditionally assessed as good. We also demonstrate the vulnerability of cooperation towards purely defecting AAs, and the inefficacy of non-discriminating cooperators in promoting cooperation. Our theoretical work contributes to identify the settings where artificial agents, even with simple hard-coded strategies, can help humans to solve a social dilemma of cooperation.