
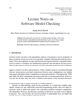
The goal of positive-unlabeled (PU) learning is to train a binary classifier on the basis of training data containing positive and unlabeled instances, where unlabeled observations can belong either to the positive class or to the negative class. Modeling PU data requires certain assumptions on the labeling mechanism that describes which positive observations are assigned a label. The simplest assumption, considered in early works, is SCAR (Selected Completely at Random Assumption), according to which the propensity score function, defined as the probability of assigning a label to a positive observation, is constant. Alternatively, a much more realistic assumption is SAR (Selected at Random), which states that the propensity function solely depends on the observed feature vector. SCAR-based algorithms are much simpler and computationally much faster compared to SAR-based algorithms, which usually require challenging estimation of the propensity score. In this work, we propose a relatively simple and computationally fast test that can be used to determine whether the observed data meet the SCAR assumption. Our test is based on generating artificial labels conforming to the SCAR scenario, which in turn allows to mimic the distribution of the test statistic under the null hypothesis of SCAR. We justify our method theoretically. In experiments, we demonstrate that the test successfully detects various deviations from SCAR scenario and at the same time it is possible to effectively control the type I error. The proposed test can be recommended as a pre-processing step to decide which final PU algorithm to choose in cases when nature of labeling mechanism is not known.