
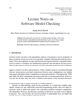
Given the common scenario where a trained model confronts significant variations in data distributions different from the training data at test time, Test Time Adaptation (TTA) has emerged as a crucial field of study. Traditional methods in TTA have focused on filtering low-entropy samples to improve model performance, primarily through entropy minimization techniques. However, these approaches exhibit limitations as they often overlook the potential classes of high-entropy samples. This oversight can result in an inadequate utilization of available data, particularly under challenging conditions where model adaptability is critical. In contrast to conventional approaches, our work diverges from the sole emphasis on low-entropy samples by leveraging the rich information contained within ambiguous samples. We demonstrate that reliance solely on entropy minimization is detrimental when dealing with ambiguous samples. To address this, we introduce Cliff, a novel framework designed to learn from ambiguous samples effectively. Concretely, Cliff comprises two innovative components: Dynamic Recognition (DR) and Gap Raising Loss (GRL). DR proposes a method for identifying ambiguous samples and dynamically assigning weights to them, enhancing the model’s focus on potentially informative discrepancies. Whereas the proposed GRL, indeed theoretically proven to be beneficial to the model, guides the model in effectively distinguishing among potential classes by emphasizing the differences in their predictive probabilities. Extensive experiments conducted on CIFAR-10-C and CIFAR-100-C datasets demonstrate Cliff’s state-of-the-art performance. Our results show an average accuracy improvement of 20.24% and 21.12% over the direct use of source domain models on target domains, respectively.