
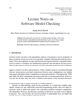
Lane detection is an important yet challenging task in autonomous driving systems. Based on the development of the Visual Transformer, early Transformer-based lane detection studies have achieved promising results in some scenarios. However, for complex road conditions such as uneven illumination intensity and heavy traffic, the performance of these methods remains limited and may even be worse than that of contemporaneous CNN-based methods. In this paper, we propose a novel Transformer-based end-to-end network, called SinLane, that attains the attention weights focusing on the sparse yet meaningful locations and improves the accuracy of lane detection in complex environments. SinLane is composed of a novel Siamese Visual Transformer structure and a novel Feature Pyramid Network (FPN) structure called Pyramid Feature Integration (PFI). We utilize the proposed PFI to better integrate global semantics and finer-scale features and to promote the optimization of the Transformer. Moreover, the designed Siamese Visual Transformer is combined with multiple levels of the PFI and is employed to refine the multi-scale lane line features output from the PFI. Extensive experiments on three benchmark datasets of lane detection demonstrate that our SinLane achieves state-of-the-art results with high accuracy and efficiency. Specifically, our SinLane improves the accuracy by over 3% compared to the current best-performing Transformer-based method for lane detection on CULane.