
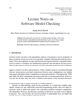
I-beams with corrugated webs have higher torsional stiffness than that of flat web beams. Furthermore, the geometrical dimensions of the beam and the web corrugation heavily influence the precision of the currently used traditional pen-and-paper methods for determining the elastic lateral-torsional buckling moment. This study aims to suggest several machine learning models with the intention of predicting the elastic lateral-torsional buckling moment of corrugated web beams. Multiple machine learning models, including Random Forests, Gradient Boosting, Categorical Boosting, and Deep Neural Networks, were deployed to develop and train models to predict the elastic critical lateral-torsional buckling moments of I-beams with corrugated web. The database used for training the different models was compiled through linear bifurcation analyses conducted on shell finite element models. The study evaluates the precision of the various machine learning models by examining their performance against statistical parameters derived from both predicted and test data. The findings from the parametric evaluation highlight the surprisingly high performance and accuracy of the machine learning models.