
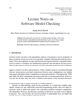
Diagnosis using time series data is an important area in medical domains. Machine learning models rely on large collections of data for successful generalisation. However, data collection in fields such as medicine is difficult, which limits the effectiveness of these models. Although techniques like data augmentation can help increase dataset sizes, they work best mainly with image data and not as well with other types of data. This is where generative models can fill this gap. We present a study of the application of a range of generative models (TimeGAN, WaveGAN, DDPM) and data inpainting models (SSSD, ExtraMAE) for time series on the domain of the classification of Inertial Measurement Units (IMUs). The aim is to assess their capabilities and the improvements obtained when used for data augmentation with different training and transfer learning methods. The results show that these methods generate synthetic data that, when added to the training data or used as pretraining data, improve accuracy. GAN methods lag behind diffusion denoising methods in generating realistic data, and are also more difficult to train. Inpainting methods obtained results similar to GAN methods but generated samples more similar to the real data and with a more stable training.